What is AI Based Testing & What are its Examples
- Serena Gray
- Jan 12, 2021
- 2 min read
Artificial intelligence (AI) and machine learning (ML) are transforming multiple sectors of the market, and impacting several elements of our daily lives.
The present state of the practice utilizes smart and autonomous agents, referred to as "test bots," to automate activities like application detection, modelling, test generation, and failure detection. A blend of machine learning methods are utilized to implement robots.
Examples of AI Based Testing strategies that have shaped over the last decade include:
Leveraging AI for web element place makes the tests quicker to create and less fragile. Furthermore, the testing community can improve the AI with the addition of new training information, alternative training procedures, more rigorous significance analysis, or new labels.
The training data is open and accessible on Kaggle, thus allowing organizations or individuals to fork the information, clean it up, add it for their proprietary evaluation frameworks, or compete to improve the classifiers.
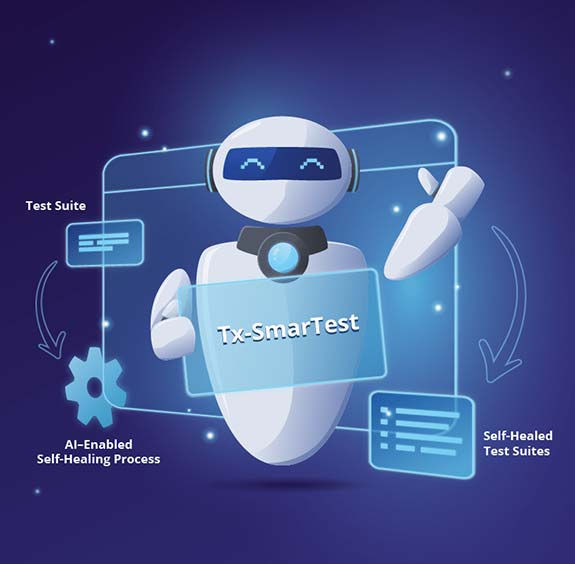
AGENT is an ML-based, multi-agent prototype for web application testing. The prototype consists of a group of intelligent agents that work in conjunction with abstract evaluation generation and net UI classification subsystems. The brokers comprise long short-term memory components trained by human testers.
For any given subsequence of measures throughout the application, the brokers predict sequences of their next possible set of actions and assertions that a human tester may make and automatically implement them onto the machine under test.
Among the trends that started this past decade, which can be predicted to last, is the use of AI to improve present tools and frameworks that target specific testing problems.
However, over time as technology progresses, and since the machines are trained on the activities of the higher-order tasks, AI is likely to take over those activities also and tackle issues that demand deeper context.
How Can We Educate?

So what can testers do to get ready for a future where AI tests applications? Step one is to determine whether you are thinking about designing and developing AI-driven systems or being an end-user of these instruments.
An engineer who's building these types of systems needs to have a fantastic understanding of AI and machine learning. Both MIT and Stanford University have freely available introductory classes on machine learning.
Various companies have open-sourced the materials for an introductory class on AI and ML abilities for the testing world, like the tools and slides used during the exercises. It's unlikely that end-users of AI-driven testing tools will need to have a deep understanding of machine learning. Vendors are most likely to provide simple interfaces and APIs for using AI-driven testing features and customizing pre-trained AI versions.
This tendency is already being followed with the advent of tools and platforms such as Google's AutoML Vision, H2O.ai, and DataRobot.
Comentários